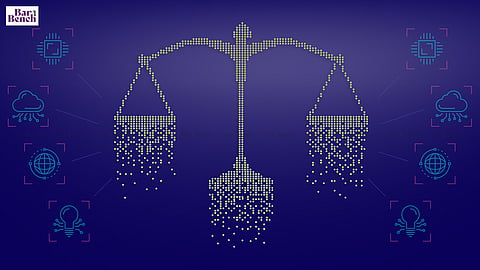
Introduction
Artificial intelligence systems have influenced all aspects of humankind pertaining to animate, virtual or abstract facts in form of data inputs, figures and codes through a set of algorithms that aid in arriving at definite solutions.
Predictive policing is the usage of analytical and mathematical techniques through gadgets in law enforcement to identify a potential criminal activity. In order to make the best use of resources or to have the greatest chance of deterring or preventing future crimes, predictive policing uses data about the locations, time, and types of past crimes to inform police strategists where they should patrol or maintain a presence.
Heralding the dawn of an era of apps and web tools supported by artificial intelligence (AI) with predictive policing growing fast, its sprawled tentacles hooking all facts and figures fed as data set inputs by investigation wings and law agencies to curb crimes, the move appears dangerous! Though appearing as an objective approach towards predictive solutions, it may prove itself as a fundamentally flawed input of promoted concepts that largely contain algorithm bias.
Criminal "Justice" amid divergent and disparate results
Introducing this technology to our overburdened legal system will assist in solutions or further complicate it, or hamper access to justice is a pertinent question. The implementation of AI systems in policing may have many undesirable consequences given the inherent power of police to detain, arrest or torture the subject accused. The technology is also labelled ‘Black box’ as these conclusions lack transparency. Actual cause behind predictive statements remains occult as citizens and law enforcement officials may not have the necessary information regarding their operational derivations. Blind reliance on such software unless in favor, shall be inevitably challenged by the accused.
In light of these factual concerns, experts must scrutinize and balance the decisions of the machine to make it credible so as to use such software to promote upholding the law of the land.
Circumstantial evidences question the absolute efficacy of the algorithms applied in predictive technologies that precede bail hearings in the U.S. where predictive technologies were implemented and validated as part of a pre-trial bail reform package. The police department of Pennsylvania uses the PredPol software, wherein collection and data processing of historical crime is accomplished and prediction of areas or regions of crime follows. Suspects at a low, medium or high risk of repeating offence in future are classified by gathering and calculating historical offence data. US Criminal Justice System uses the App COMPAS (abbreviated for Correctional Offender Management Profiling for Alternative Sanctions) for assessing risk to determine the terms of parole for the accused in question. Since minority population is usually targeted, this big data is already biased.
China’s predictive policing officiated arbitrary detainment of people in Xinjiang. In a case of the Wisconsin Supreme Court in the United States, COMPAS software was employed on the petitioner obviating the due process, thus violating his rights. Despite the Supreme Court verdict in favor of the State, the judges held that prior warning of needs of usage of these tools have to be given.
JARVIS, an AI-powered 700-camera-loaded video analytics solution, installed in 70 jails of Uttar Pradesh, predicts suspicious behavior of prisoners by analyzing their body language. Active diffusing of crime and plots is the purpose. But is this measure proportional in magnitude to the prime objective? Continuous monitoring of inmates specially females is embarrassing, challenging their right to privacy. Thus, violent behavior and psychosomatic changes are expected consequences.
The "black-box" contradiction results in a system that is confusing and complicated. The outputs of the AI algorithms cannot be questioned or challenged since these are protected by intellectual property law. Furthermore, for these AI algorithms to function, the software must interact with large data sets produced using a variety of characteristics that might not directly relate to the crime. Consequently, the decision-making process frankly loses impartiality, and right to a fair trial is violated. Additionally, the individual subjected to the outcomes of these AI tools may not even comprehend the rationale behind the algorithm's judgments, as these are absolutely arbitrary when depended upon.
Indian Magistrates face challenges in handling critical issues occurring in pre-trial stages like issuing search warrants, granting remand/bail, video conferencing for confessions, etc. Various factors hindering judicial processes have been huge backlog of cases, caste biases and lax attitude towards safeguarding the rights of the accused. Here, machine and its ideas may overpower human intellect when it comes to raising a reasonable suspicion and even questioning police investigations. (except those ordered under Section 156(3) CrPC) In their article titled ‘Is Big Data Challenging Criminology?’, Moses and Chan clearly depict that judicial officers may take the AI data as sufficient input for decision-making and may become oblivious to understanding AI software deluge and the turbulence it might create.
Indian procedural criminal law allows Magistrates to get involved at crucial pre-trial moments, like issuing search warrants, giving detention or bail, and recording confessions. However, obstacles preventing Magistrates from successfully applying their authority to intervene at this point include implicit caste prejudices, a protracted backlog of cases, and careless attitudes about defending the rights of the accused. If presumptive investigative outputs of predictive policing software might be acceptable by law enforcers without much speculation, the situation will be further aggravated when the results of predictive software are used to support reasonable suspicion.
The possibility that law enforcement organizations may employ such technology to replace established traditional police methods rather than merely enhancing them is a key issue with the use of predictive policing. Predictive software is used in the US to determine whether to make an arrest based on risk assessments that take into account the likelihood of crime. The "reasonable suspicion" threshold established by the U.S. Supreme Court in Terry v. Ohio (1968) governs Fourth Amendment arrests (1967). According to it, to justifiably stop a person, the police must be able to “point to specific and articulable facts which, taken together with rational inferences from those facts, reasonably warrant that intrusion.” This criterion is similar to Section 41(1)(b) of the Code of Criminal Procedure, 1973 (the "CrPC") in India, which gives police the authority to make arrests where there is a reasonable suspicion that a person has committed a crime that is punishable by law. Police personnel must have concrete data in hand to assess before acting on such a suspicion. The basic right against arbitrary arrest and imprisonment is advanced by compliance with Section 41 CrPC, as is evident from the Apex Court's reasoning in Arnesh Kumar v. State of Bihar (2014). Now, predictive policing may allow law enforcement officers to develop "reasonable suspicion" by providing concrete information (such as risk assessment scores) from which conclusions may be derived regarding whether to make an arrest. There is a substantial evidence showing how data analytics simply reinforce social prejudices and fall short of providing explanations for specific occurrences, casting doubt on the accuracy of predictive algorithms. The Supreme Court of Western Australia in DPP v. Mangolamara (2007) doubted authenticity of reports produced by instruments that predicted recidivism before sentence. Court said that the prediction algorithms lacked the necessary social context for minority, aboriginal communities and other marginalized socioeconomic strata yielding inaccurate results. In light of the fact that these automated tools did not constructively take into account the socioeconomic situations of the individuals being profiled, the Court concluded that standards of evidence indicated a sort of "knowledge based on experience" and did not inspire sufficient trust.
How Predictive Policing may be Employed, constructively
Use of predictive policing must be transparent and accountable with a dual approach essential to chart out its constitutional implications. Far-reaching consequences as in cases of criminal law are a concern as algorithmic case-based decisions fail to identify situational and circumstantial evidences disfavoring the accused without due process. Well! again we humans have to find solutions. Public engagement and encouraging community participation by the formation of citizen review boards, consulting experts on data audits, incorporating views and decisions of the most learned faculty, introducing this subject in various faculty courses, linking big databases across globe, holding seminars and conferences, and finally ensuring democratic accountability are way forward to systematize this dynamic digital devisor to improve its viability and overall acceptability.
A logical analysis would conclude that use of predictive policing algorithm does not predict future events, only the statistical risk of their occurrence be derived out. The actual benefit of predictive policing lies in situational awareness, i.e. information necessitating action upon what the software considers or declares as ‘risk’ factors. Accordingly, these AI tools should carry sophisticated software based on the magnitude and scale of operations and should cover all arenas under the jurisdiction of a department where the case story is served as input data. Also, there must be a compulsory revelation of the use of software by prosecutors and the police.
The inevitability and pervasiveness of bias in big data makes it essential for civil society and intellectuals to constantly scrutinize such claims, subjecting it to democratic analysis. In predictive technologies, internal oversight mechanisms can be installed, like ‘privacy by design requirements’ foisted by robust regimes for data protection, ensuring functional oversight. The need of the hour is to subject these predictive technologies to democratic consultations, as their impact on marginalized communities appears to be uncertain and probably oppressive truly indicated by the Status of Policing in India Report (2019) that police personnel have a significant bias against the Muslim community, migrants and illiterates. Consequently, the data-driven predictive software exhibits encoding patterns that depict discriminatory behaviour by police. This spreads a wrong message in a secular country like India where the sacredness of our constitution is challenged and hence there are biases in justice delivery.
The above discussion necessitates evaluation of effects of predictive policing and implement policies to mitigate the harmful consequences this intrusive technology might have on human rights. Data assimilation is a mammoth task and its customized application is herculean. The aim is to maximize the advantages and make it trustworthy.
The author, Kartikeya Kothari, is a second year law student at Maharashtra National Law University, Mumbai.