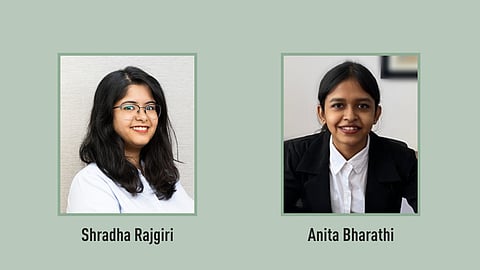
Artificial Intelligence (AI) has made its entry in all fields, including the healthcare sector. The impact of such machine learning innovation, in comparison to other industries, is more impactful and conspicuous amongst medical devices.
However, the advanced technology that often overrides human intelligence also carries with it a lot of questions that are yet to be addressed by the law. One such uncertainty is the determination of liability when AI-induced machines and technologies commit errors, resulting in radical miscalculations in analysis.
The first of this two-part article aims to address how such AI-induced medical devices (AIMD) are legally recognized and the lacunae in Indian laws with respect to determination of liability.
With growing developments in the field of technology, AIMD can help predict, diagnose and manage the well-being of a patient. This innovation can help simulate cognitive human intelligence and can have a number of applications across the healthcare industry. It is, however, imperative to first understand the functioning of AI in the medical field at large and machine errors committed by AI. Any such errors or miscalculations ought to be addressed by having a framework to determine legal accountability.
Legal recognition of AIMD
In the United States, various AIMDs have been patented under the Patent Act (35 U.S. Code), under which many medical technologies that assist in the discovery of medicines are also patented. The United States Food and Drug Administration (FDA) is the approving authority for medical devices and has a regulatory mechanism to check the quality and safety of the machines.
The FDA is tasked with certifying the safety and efficacy of many AI-driven medical products. The agency largely regulates software based on its intended use and the level of risk to patients if it is imprecise. If the software is intended to treat, diagnose, cure, mitigate, or prevent disease or other conditions, the FDA considers it a medical device. The US government has also laid down certain exemptions on what kind of software are to be excluded from the definition of a ‘medical device’.
Furthermore, the International Medical Device Regulators Forum (IMDRF) in 2021 released its proposed document elaborating on Machine Learning enabled Medical Devices – A subset of Artificial Intelligence–enabled Medical Devices. The document deals with the scope, terms and definitions to be used while making references to AIMD. The United States of America, the European Union, Singapore, Australia and China are participants of the IMDRF.
In India, on the other hand, AIMD has been recognized through the Medical Devices Rules, 2017, which identifies ‘software’ as a medical device by expanding the scope of the definition of “drug” under the Drugs and Cosmetics Act, 1940. An amendment was brought to the Rules in 2020 by which all medical devices excluding 37 specific categories such as nebulizers, BP monitoring devices, CT scan equipment, etc, are to be registered mandatorily with the Central Licensing Authority. Various tests are positioned in the form of registration and a certificate board that would check the efficiency and safety of the said devices. The obligation for medical devices manufactured or imported in India is on the importer or manufacturer of the device.
In a recent ruling, the US District Court for the Eastern District of Virginia (Alexandria) held that AI does not qualify as a ‘person’ in order to be legally recognised. This further questions the likelihood of AI being held liable for its errors. Though the extent of AI involvement differs in each device, its existence cannot be ignored since AI is crucial for the functioning of those devices. Moreover, the registration and certificate procedure as laid down in the Medical Devices Rules, 2017 do not ensure permanent safety of those devices; it rather lays down a grievance redressal mechanism through which malfunctions or complaints can be tackled.
Black box algorithms as a roadblock in med-mal claims
Medical malpractice (med-mal) systems have so far helped in understanding and determining liability when a claim is made. They are legal claims made by or on behalf of a patient when a case of medical negligence or malpractice transpires. However, med-mal lacks any provision to address the claims when AI is involved. Black box algorithms are present in all AI medical devices, including the ones that require human efforts to perform. A black box algorithm (also referred to as ‘model of the device’ alone) does not include inputs and outputs. They are known for their probability-based results as the only logic behind the model. Here, the processing that takes place within the device is hidden even from its builder, making it nearly impossible to trace the source of any mistake that occurs.
Various AI medical devices have been analyzed through this lens, although none of them were constructive or pragmatic. Even the maximum transparency that could be achieved by these studies is when the processing is reduced to mathematical calculations or algorithms, which are, however, only understood by its builders, thereby rejecting the chances of a layperson understanding the functioning of an AIMD. To streamline the models, researchers suggest that the efficiency and safety of an AIMD can only be observed by randomized clinical trials showing positive results. Such results would thereafter be compared to human made results to test the accuracy.
An example of a solution to this issue is Corti Orb, a software developed by a Danish company to identify cardiac arrests when placed on a desk, by way of observing movements. Corti uses black box algorithms to produce results, proven to be 93% accurate. However, possible error or false alarms by the machine have triggered debates questioning the credibility of the machine, and have complicated the determination of liability while blurring the possibility of the creator being held liable.
Apart from the knowledge aspect in determining liability, AIMDs are also required to combat other glitches arising in a non-AI medical device requiring 100% human effort. Placing both AI and non-AI medical devices together under the head of ‘medical devices’ pitches further complications to the determination of liability. When non-AI medical devices such as bone cements, surgical dressings, umbilical tapes, etc lack quality or safety as required under the rules, the manufacturer will be the only person liable. This would apply to situations where such devices have been successfully used in the treatment of patients and an inconvenience occurs at a later point of time.
This approach has been undertaken by a US Court, in a case where a medical device called ‘Pinnacle’ was implanted successfully by a medical practitioner, but later on caused health complications to the patient. The Court held the device to be defective and the manufacturing company strictly liable. It could be inferred that the Medical Device Rules has brought in a similar approach in handling failure of medical devices which includes AIMDs, even though the Indian courts are yet to decide any matter on these lines.
Conclusion
With the very limited options regarding black box algorithms, there is nothing beyond accurate calculation and output that can be expected out of the same. Since breaking down black box algorithms into understandable formats is not possible, any wrong calculations by the machine error are hard to identify unless or until the concerned persons raise a doubt. The chances of such doubts arising in itself is a question, since AI is run purely by the previous results and probabilities.
In the absence of any law covering such technological aspects in India (with the Data Protection Bill 2021 being withdrawn recently), there is no method available to investigate the protection afforded by black box algorithms, further hindering the determination of liability.
Shradha Rajgiri and Anita Bharathi are Senior Associate and Associate respectively, with Shivadass & Shivadass (Law Chambers).